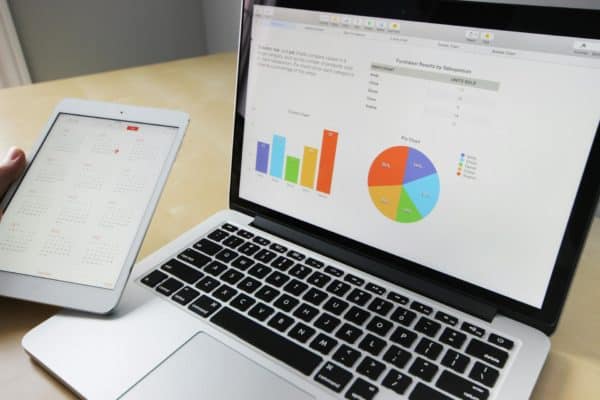
In the ever-evolving landscape of data science, staying abreast of the latest methodologies is imperative for professionals aspiring to remain at the forefront of innovation and relevance. As we delve into this discourse, we shall unravel the intricacies of the most prominent techniques shaping the data science landscape in 2024. Whether you are a seasoned professional seeking to refine your skill set or a novice embarking on your journey in this dynamic field, this discourse aims to furnish you with invaluable insights in navigating the challenges and opportunities that lie ahead.
Data science remains pivotal in our data-driven world, with increasing demand for skilled professionals. Pursuing a data science course in IIT provides rigorous academic training and equips individuals with practical skills demanded by the competitive job market. With a cutting-edge curriculum curated to meet industry standards, students gain proficiency in statistical analysis, machine learning, and data visualization. Furthermore, access to state-of-the-art resources and experienced faculty fosters a conducive learning environment. Armed with this comprehensive education, graduates are well-positioned to secure lucrative positions in data science, navigating the complexities of the modern job market.
What Is Data Science?
Data science is a multidisciplinary field that utilizes scientific methods, algorithms, and systems to extract insights and knowledge from structured and unstructured data. It amalgamates various techniques from statistics, computer science, and domain knowledge to analyze vast datasets and uncover valuable patterns, trends, and correlations. Data scientists employ machine learning, data mining, and visualization tools to derive actionable insights, aiding decision-making processes across industries. By harnessing the power of data, data science drives innovation, enhances business strategies, optimizes processes, and enables predictive modeling, ultimately empowering organizations in making informed decisions to gain a competitive edge in today’s data-driven world.
Importance of Data Science
Data science is pivotal in today’s data-driven world by extracting meaningful insights from vast and complex datasets. It allows businesses in making informed decisions, optimize processes, and drive innovation. Through advanced analytics techniques like machine learning and predictive modeling, data science helps businesses patterns, identify trends, and correlations that would otherwise remain unidentified. This empowers companies to enhance their products and services, personalize customer experiences, and improve operational efficiency. Moreover, data science fosters evidence-based decision-making, enabling businesses to stay agile and competitive in rapidly evolving markets. Data science catalyzes driving growth, efficiency, and innovation across industries.
Data Science Techniques
Data science techniques encompass diverse methodologies and tools used to extract actionable insights from data. These techniques include data preprocessing, exploratory data analysis, statistical modeling, machine learning, and data visualization. Data preprocessing involves cleaning and transforming raw data for analysis, while exploratory data analysis uncovers patterns and relationships within datasets. Statistical modeling employs mathematical algorithms to make predictions or infer relationships. Machine learning algorithms learn from data to make predictions or decisions without explicit programming. Data visualization presents findings in a visual format for easier understanding and interpretation.
These are the popular data science techniques:
Regression: Regression is a statistical method used to analyze the relationship between one dependent variable and one or more independent variables. It aims to understand how changes in the independent variables affect the dependent variable. Regression models can be linear or nonlinear and are commonly used for forecasting and predicting continuous outcomes.
Classification: Classification is a supervised learning technique where the goal is to categorize data points into predefined classes or labels. It involves training a model on labeled data to learn the relationship between input features and output labels. Common classification algorithms include logistic regression, decision trees, support vector machines, and neural networks. Applications range from spam detection to medical diagnosis.
Clustering: Clustering is an unsupervised learning technique used to group similar data points together based on their characteristics or features. Unlike classification, clustering does not have predefined classes; instead, it discovers inherent structures within the data. K-means clustering, hierarchical clustering, and DBSCAN are popular algorithms for clustering tasks, which find applications in customer segmentation, anomaly detection, and image processing.
Anomaly Detection: Anomaly detection is also popularly known as outlier detection that involves identifying patterns in data that deviate significantly from the norm. Anomalies can signify critical events or errors in the data. Techniques for anomaly detection include statistical methods, machine learning algorithms such as isolation forests or one-class SVMs, and unsupervised learning approaches like clustering. Anomaly detection is crucial in fraud detection, network security, and fault detection systems.
Data Visualization: Data visualization is the graphical representation of data to communicate insights and patterns effectively. It involves creating visualizations such as charts, graphs, and maps to explore, analyze, and present data in a meaningful way. Data visualization enhances understanding, aids decision-making, and enables stakeholders to grasp complex information quickly. Popular visualization tools include Matplotlib, Seaborn, Tableau, and D3.js.
Predictive Analytics: Predictive analytics involves using historical data, statistical algorithms, and machine learning techniques to forecast future trends, behaviors, or events. It aims to predict outcomes based on past patterns and relationships in the data. Predictive analytics finds applications in various domains, including sales forecasting, demand planning, risk assessment, and healthcare outcome prediction.
Statistics: Statistics forms the foundation of data science, providing methods and techniques for collecting, organizing, analyzing, interpreting, and presenting data. Statistical concepts such as probability distributions, hypothesis testing, and regression analysis are fundamental in understanding data and making data-driven decisions. Statistical principles guide data scientists in designing experiments, drawing conclusions, and inferences from data.
These data science techniques empower analysts and scientists to extract insights, make predictions, and derive value from data across diverse domains and industries.
Conclusion
Mastering popular data science techniques in 2024 is paramount for professionals aspiring to thrive in the dynamic landscape of analytics. Pursuing a data science course in IIT is a pivotal stepping stone towards a lucrative career. With a curriculum meticulously crafted to align with industry demands and access to cutting-edge resources and renowned faculty, IIT equips students with the knowledge, skills, and practical experience necessary to excel in the competitive job market. The rigorous education offered at IIT prepares individuals for the challenges of today and positions them for success in the ever-evolving realm of data science.